Laurent Dinh
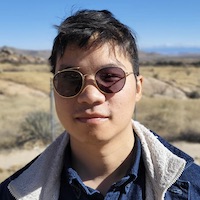
I am a machine learning researcher. I have been mainly working on deep generative models, variational models and most notably flow-based models, and (mis)understanding of machine learning methods. I also follow work in connected areas like probabilistic modelling, approximate inference, optimization, learning of discrete structures, and sociotechnical studies of machine learning.
I obtained my PhD in deep learning at Mila (Montréal, Canada), under the supervision of Yoshua Bengio. Prior to that I studied at École Centrale de Paris (Paris, France) in applied mathematics and at ÉNS Paris-Saclay (Paris, France) in machine learning and computer vision. I had the privilege to work in the machine learning group led by Nando de Freitas both at UBC (Vancouver, Canada) and DeepMind (London, United Kingdom), and also at Google Brain (Mountain View, US), under the supervision of Samy Bengio.